ZAPRASZAMY DO UDZIAŁU W NASZYCH PROJEKTACH
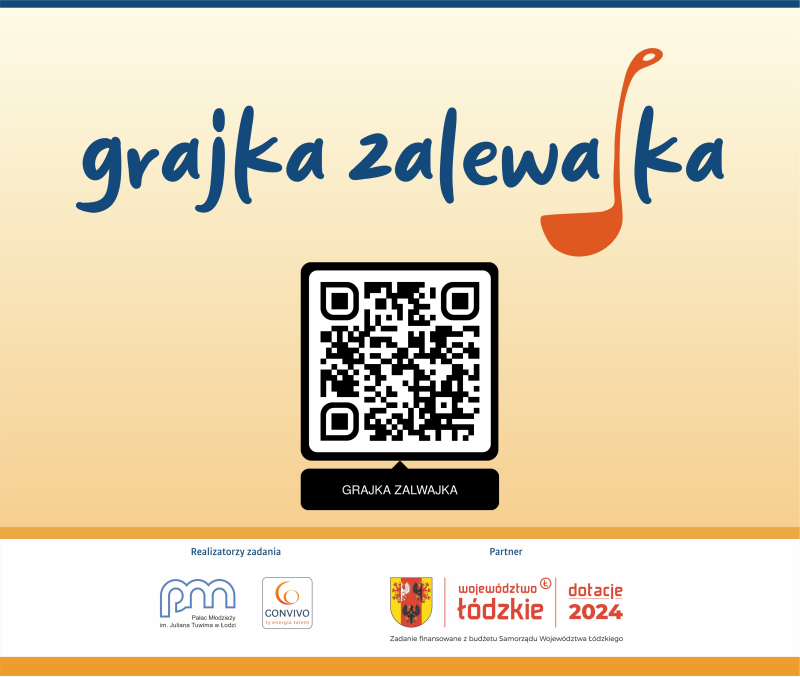
CONVIVO DLA... - stałe programy Fundacji
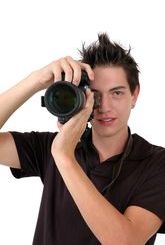
DLA CIEBIE
Jesteś młodym utalentowanym twórcą, artystą, masz wiele pomysłów na prezentację Twoich umiejętności artystycznych, szukasz wsparcia w rozwijaniu Twoich zdolności? Zainteresuj się naszym programem opracowanym specjalnie dla CIEBIE !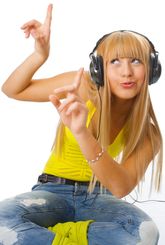
DLA WAS
Śpiewasz, grasz, tworzysz w zespole muzycznym, wokalnym, tanecznym, teatralnym lub innym o charakterze kulturalno-artystycznym? Szukasz wsparcia dla Waszego zespołu? Zapraszamy WAS do współpracy!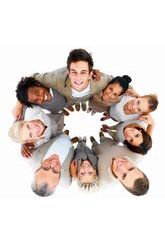